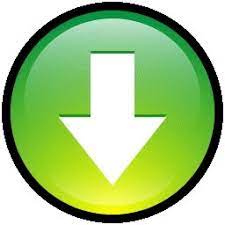
Secondly, due to the data complexity, it is challenging to differentiate the incorrect predictions from the correct ones on real-world large-scale datasets. Firstly, correct predictions are generally dominant over incorrect predictions.
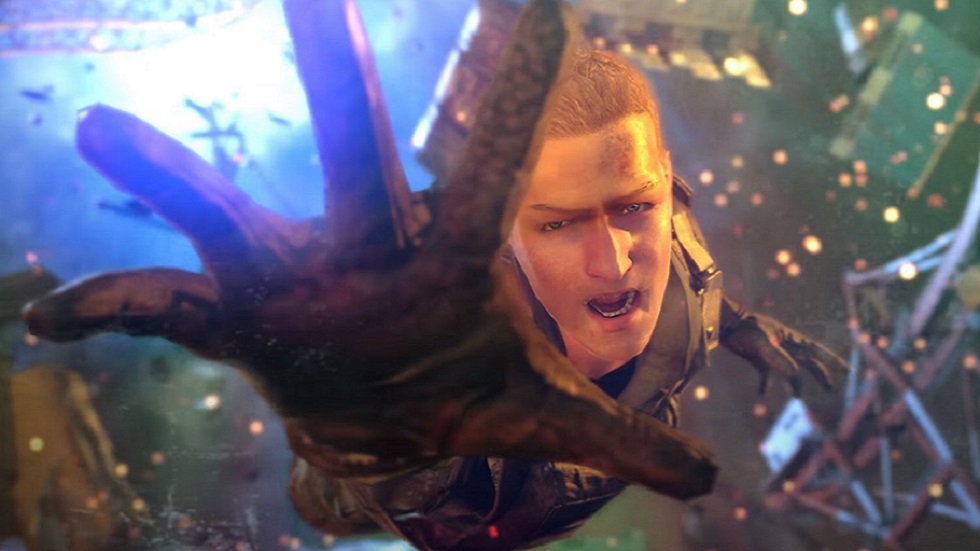
In such a setting, we observe that the trustworthiness predictors trained with prior-art loss functions, i.e., the cross entropy loss, focal loss, and true class probability confidence loss, are prone to view both correct predictions and incorrect predictions to be trustworthy. In this work, we study the problem of predicting trustworthiness on real-world large-scale datasets, where the task is more challenging due to high-dimensional features, diverse visual concepts, and a large number of samples. Prior efforts have been proven to be reliable on small-scale datasets.
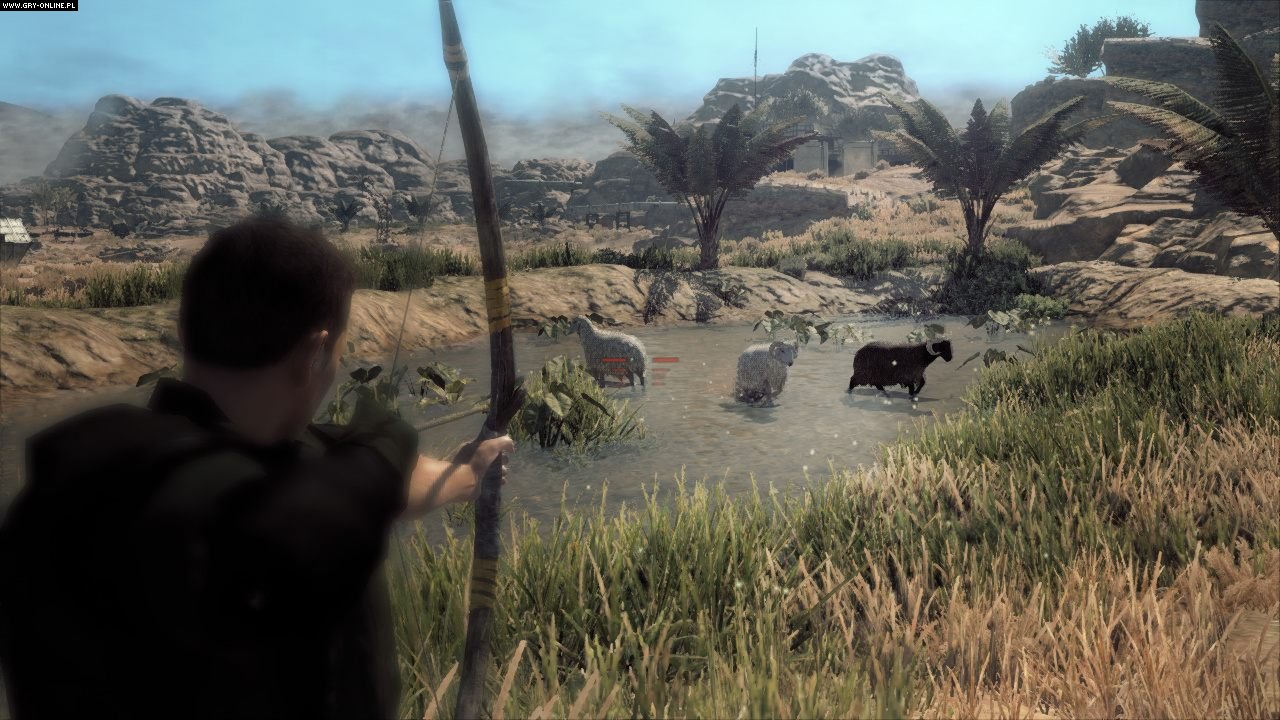
Abstract: Understanding the trustworthiness of a prediction yielded by a classifier is critical for the safe and effective use of AI models.
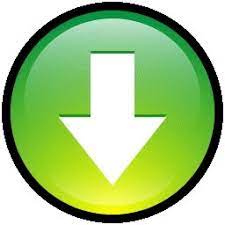